Technology and trust – using data and AI responsibly in healthcare
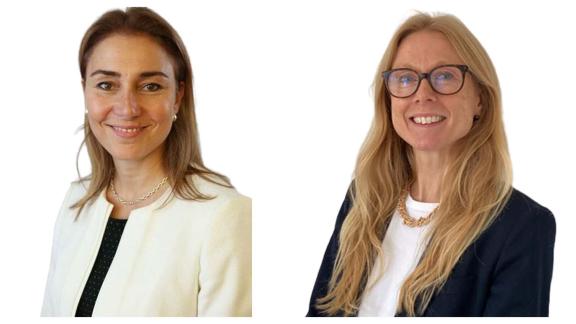
Public concerns around data use could take away from the huge benefits that technology can offer to accelerate health research and develop new treatments, say Dr. Angela Spatharou and Kate Robinson of IBM.
IBM is supporting NHS ConfedExpo in Manchester on 14 and 15 June
Trust is one of the foundational principles of healthcare. It is critical when discussing the handling of healthcare data or the application of artificial intelligence (AI), that we place a strong emphasis on trust to guide how we operate.
The recent explosion of ChatGPT and AI, the increasing use of data-driven analytics and some of the headlining data breaches, have all created high levels of public attention around where technology is taking us and how much control we have in the way it is actually used. The conversation is no longer just about ‘your life in their hands’, but also ‘your data in their hands.’
Trust is an activity of both head and heart
These concerns could derail the huge benefits that technology and the use of our unrivalled NHS data can offer us, to be able to accelerate health research and develop new treatments, better care pathways and services. It is, therefore, critical, that we use trust as a core design principle as we consider how we use this technology.
Trust is an activity of both head and heart. When it comes to data management, there are a number of underpinning foundations that need to be in place in order to build trust and confidence:
- People need to feel they have control and are engaged transparently and honestly around how their information is being used.
- People need to know data is managed according to the established Caldicott principles of confidentiality, in order to both guard and also to share information appropriately, whether for the benefit of the individual or that of the wider population.
- People also need to know this is regulated by an established external body, with clear standards and a mandate to impose meaningful sanctions.
- There needs to be evidence that the outputs of the data analytics stand up to scientific inquiry, peer review and professional consensus.
- People tend to place their trust in organisations and individuals that they perceive are trustworthy. This can include trusted professional, public or private organisations with a track record of treating data securely to protect it from attack and compromise, and who take their data guardianship responsibilities seriously.
AI, and indeed other kinds of advanced analytics, require more expansive thinking. AI has far surpassed its initial ambition to automate simple repetitive tasks requiring low-level decision-making, and has rapidly grown in sophistication, thanks to more powerful computers and the compilation of huge data sets, and to machine learning.
Five safety tests for AI software
We are now at a point where foundational models have consumed and analysed massive amounts of data and use generative algorithms to create responses that have natural language feeling. This step change requires careful consideration, if we are to use this technology responsibly to deliver benefits in healthcare.
We believe there are five tests that should be applied before an AI software should be trusted and applied in safety critical environments like healthcare.
- The resulting analytics should be robust, that is to say, give accurate, valid and repeatable answers. The integrity of the training data should be protected from attack or from being compromised.
- These systems should be fair. Bias, although always present, should be managed and measured and allowances should be made to ensure the benefits of these systems are truly representative of the whole populations that they serve.
- The system should be as explainable to the user as possible. While we acknowledge this can be complex, for example in neural networks that may be many layers deep, there is no place for ‘black box’ techniques where the workings are obfuscated. We are actively working on approaches to make things as explainable to human beings as possible.
- AI should be transparent, with companies being clear about who trains their AI systems, what data was used in training and, most importantly, what went into their algorithms’ recommendations.
- The data handling that drives the AI should be held to the highest standards, to ensure that our most sensitive personal data is kept private and safe.
The public need to be able to trust in the processes and in the organisations involved to support the ongoing digital modernisation of healthcare, so that it can achieve its promise.
AI offers the opportunity to augment human ability, not to replace it
It is for that reason that IBM has launched watsonx.governance: a toolkit and overarching framework that uses a set of automated processes and methodologies to help us manage AI use with confidence.
The debate requires a mature, balanced discussion to get people to think about the use of their data in more complex terms than ‘good’ or ‘bad’, encompassing both concerns and benefits, risks and mitigations.
AI offers the opportunity to augment human ability, not to replace it – and in healthcare terms, we need to understand what it means for health professionals to operate at the ‘top of their augmented licence.’
The greatest risk of AI and technology in healthcare is not that they take over, but rather that we fail to harness their potential to solve the major challenges the health and care system is facing today.
Dr. Angela Spatharou is UKI and EMEA healthcare and life sciences leader at IBM Consulting.
Kate Robinson is managing director, NHS, at IBM Technology.
You can follow Angela and Kate on LinkedIn: